Summary
- Committing to improving health equity means collecting and stratifying data to identify inequities, help set priorities, and drive improvement activities.
IHI's Improving Health Equity: Build Infrastructure to Support Health Equity guide provides examples of how organizations have built the infrastructure to improve health equity. Each strategy includes a brief description, key recommended actions, examples of specific changes that organizations tested for each action, challenges and mitigation strategies, lessons, and additional tools and resources. This excerpt describes one of these strategies.
A health equity improvement strategy requires data collection and stratification to identify inequities, help set priorities, and drive improvement activities. This strategy applies to numerical performance data for clinical processes and outcomes, patient experience, and public health. These data typically are summarized in measurement dashboards and scorecards appropriate to different levels of a health system. We also refer to REaL data — attributes of race, ethnicity, and language (REaL) tied to individual data records — used to stratify clinical, patient, and public health measures.
The Improving Health Equity: Build Infrastructure to Support Health Equity guide includes examples for collecting and using REaL data to improve health equity, mostly focused on stratification by race and ethnicity data, with relatively little focus on language data. However, the data concepts and recommendations are relevant to all demographic factors that may be associated with inequities in care, including sexual orientation and gender identity (SOGI). As health care organizations obtain and steward additional demographic data for the people they serve, it will be critical to stratify data across multiple factors (e.g., race and ethnicity and language and gender identity).
To create a data infrastructure, organizations need to provide staff with training and support in obtaining accurate REaL data; understand why they want to stratify data by REaL factors; characterize missing REaL data; and assess the accuracy of data.
Understand Equity Data Basics
In 1997, the US Office of Management and Budget (OMB) required reporting on race and ethnicity by federal agencies and beneficiaries of federal dollars. The OMB developed standardized categories for race and ethnicity and as a result many health care organizations adopted the OMB categories. Additionally, the OMB categories for race and ethnicity are included in all electronic health records. OMB standards also enable individuals to select multiple racial categories.
A 2014 Health Research and Educational Trust publication summarizes dimensions of valid REaL data:
- Accuracy: Self-identified, correctly recorded, consistent categorization?
- Completeness: REaL data captured across all services? Percentage unknown, other, or declined tracked and evaluated?
- Uniqueness: Are individual patients represented only once?
- Timeliness: Are data updated regularly?
- Consistency: Are data internally consistent? Reflect the patient population served?
We explored several of these dimensions in the Pursuing Equity initiative and expand on these in the next sections. [Note: During the Pursuing Equity initiative, eight US health care organizations used the IHI Framework for Health Care Organizations to Improve Health Equity to identify and test specific changes to improve health equity.]
Provide Staff Training and Support in Obtaining Accurate REaL Data
In order to stratify, characterize, and assess REaL data organizations must first develop a data collection plan. Pursuing Equity organizations found it necessary to train and support staff in consistently interacting with patients to collect REaL data.
Examples of changes tested:
- HealthPartners in Bloomington, Minnesota, provides a common script for staff: “It is important that we are able to identify any health-related issues you may be at risk for based on your race, ethnicity, or country of origin so we can provide you with the best care. This information will remain confidential.”
- Henry Ford Health System (HFHS) in Detroit, Michigan, provides patients with a brochure, “We Ask Because We Care,” available in Arabic, Spanish, and English. The brochure explains why the health system requests REaL information, emphasizes that all patient information is confidential, and describes how the information will be used in quality improvement efforts to eliminate disparities in health care.
Articulate the Reasons for Stratifying REaL Data
All Pursuing Equity teams derive their race and ethnicity categories from the OMB categories and may also include more granular categories that roll up to the standard OMB categories. Organizations have a variety of reasons for stratifying performance indicators using REaL factors.
Pursuing Equity teams stratify REaL data at their organizations to:
- Identify where inequities exist in order to target quality improvement initiatives to reduce gaps between groups;
- Understand the demographics of the community served by the organization;
- Satisfy requirements in grant applications and for potential funders;
- Better align the health care workforce composition with the community served;
- Meet contractual compliance obligations;
- Provide and manage interpreter services.
Example of changes tested:
- Henry Ford Health System uses the OMB categories to stratify their data by ethnicity. To better serve their patients, the health system gathers granular origin data to reflect community demographics. Figure 2 displays a page from Henry Ford’s brochure, “We Ask Because We Care.” Question 2 includes a category for Arab or Chaldean identity since there is a large Arab population in Detroit.
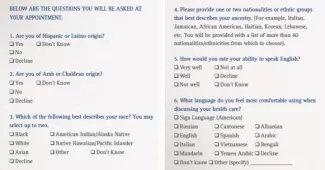
Figure 2. Henry Ford Health System REaL Data Collection Example
Characterize Missing REaL Data
In many organizations, identifying and reducing missing REaL data is a typical improvement project. Segmenting rates of missing REaL data by region or assigned primary care home is a starting step to determine opportunities for improvement. As with other patient-provided information, incomplete REaL data are likely to vary by mode of collection (e.g., in person, mail, patient portal). Pursuing Equity organizations estimate that there are higher rates of missing REaL data for ambulatory care patients than for hospital inpatients.
Discussions with Pursuing Equity teams suggest that 5 percent of patients with missing race or ethnicity categories is an achievable target. Even lower rates for missing language preference data appear possible. As missing data rates increase above a 5 percent threshold, clinicians and staff may have increasing questions about the validity of data displays and analysis that stratify by REaL factors, which can stall improvement efforts.
In every health care organization, some patients will have missing data for one or more REaL factors. Organizations with low rates of missing REaL data generally exclude these patients from data analysis and displays with minimal risk, other than loss of precision in estimates. On the other hand, if missing REaL data occurs more frequently among some groups than others, like patients with a specific diagnosis or condition, simply ignoring the patients with missing REaL data can bias the summaries.
For example, what should you do to more deeply understand a population of patients with diabetes, with a focus on racial disparities in current HbA1c test results less than 8.0? If analysts provide summary data that includes the group with no race reported, reviewers can see for themselves whether further analysis is needed. The example data summary shown in Table 1 allows the reviewer to make a rough check: If all the patients with no reported race were assigned to either the black population or the white population, would the message in the summary change?
If the message changes, then you will need to dig deeper before drawing conclusions or launching interventions.
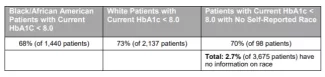
Table 1. Example Data Summary Showing Percent of Diabetes Patients with Self-Reported Race
In this example, assigning the 98 diabetes patients with no self-reported race to either the black or white groups does not change the message that black patients show a lower rate of HbA1c control than white patients; the reported percentages by race, to two digits as the original table, do not change no matter how you assign the 98 patients to the black or white groups.
It is also possible to use specific analytic methods to account for missing race and ethnicity data. A sophisticated approach, originally developed by RAND analysts, uses surname analysis and geocoding to impute race and ethnicity for patients with missing information.
Example of changes tested:
- California’s Kaiser Foundation Health Plan and Hospitals (Kaiser Permanente) has been using self-reported REaL data obtained directly from Kaiser members for the past three years. As of January 2019, almost 90 percent of its more than 12 million members have self-identified race and ethnicity data in Kaiser’s data warehouse. Some subsets of patients are close to a 3 percent level of missing REaL data, enabling Kaiser analysts to reduce or eliminate reliance on imputation in reports that stratify performance measures by race and ethnicity.
Assess the Accuracy of Your REaL Data
Best practice for REaL data requires self-identification by the patient or patient proxy, with the implication that the patient’s choices will provide the most accurate records. As with any data item, organizations need REaL data quality assurance to ensure that REaL categories indicated in the data records accurately match patient choices.
Starting points for any data quality assurance program include the following:
- Validation sampling: Randomly select a sample of patients for an additional interview or interaction to inquire about REaL categories and compare to recorded REaL information. Consult with your quality or information systems experts to create an appropriate sampling plan and analysis that will serve your needs.
- Observation of patients: How well do patients understand what is being asked with regard to REaL data? Start with five patients to ask, “What can we do to make it easier to respond to our questions about race, ethnicity, and language use?”
- Observation of staff: How well do staff present the request for patients to respond to the REaL choices? Does each encounter or exchange follow your organization’s protocol? As few as five observations can indicate lack of consistency in following a procedure or protocol.
Examples of changes tested:
- Main Line Health in Pennsylvania conducts quarterly quality assurance on REaL data collection by auditing in-person and telephone patient registration.
- HealthPartners in Minnesota reviews REaL data collection rates annually to look for deterioration from baseline performance.
- Partners HealthCare in Boston conducted a special survey study, sponsored by their Health Equity and Quality Committee, to assess accuracy of REaL data. The study involved a random selection of 1,000 patients, across multiple sites, who had REaL data noted in their records. These patients were contacted by telephone to verify their REaL data, with oversampling of patients of color at multiple sites to account for potential higher non-response rates from this population. Responses were compared to fields in the health system’s data records as a check on REaL data quality.
For health systems undergoing a merger or acquisition, REaL data categories in legacy information systems are likely to differ; these organizations should expect to invest time and staff effort to align and potentially rebuild patient data in the merged information system.
To learn more, download the free Improving Health Equity: Guidance for Health Care Organizations guides.